AI is not just about fancy robots. AI can also be effectively used in workplace investigations involving complex employee issues.
Workplace problems with employees stem from many types of misconduct like harassment, discrimination, inter-personal conflicts, performance, and bullying. Workplace issues are common and yet costly. For example, in 2018, the Equal Employment Opportunity Commission (EEOC) filed almost 14% more sexual harassment lawsuits than in the previous year. The result was around $56.6 million in compensation payments to victims. Other areas of workplace problems such as race and age discrimination cases have also seen an uptick in lawsuits. Cases involving employee ‘retaliation’ (for example, in whistleblowing cases) have witnessed the greatest increase among litigation cases.
Complex workplace situations often require the discovery and analysis of massive amounts of digital data. But employee monitoring and investigations is also a highly sensitive area. The application of artificial intelligence (AI) to the investigation of employees can help an organization to skillfully navigate these tricky situations and find evidence to manage the fallout.
Tricky situations in the workplace
The modern workplace is a data-rich environment. According to NetSkope’s 2019 Cloud Report, on average, an enterprise uses 1,296 cloud services. The data associated with these services exist in a complex network of people and devices. This data can offer deep insights into behavioral patterns, show evidence of communications, data leaks, and much more. If a complicated and sensitive issue arises at work, the data generated by employees can be used to manage and potentially offer proof of the underlying problems.
But what type of situations can benefit from the use of AI to provide actionable insights from data?
Working with the enemy: Interpersonal issues and harassment
There are often two sides to a story. The more complicated that story is, the more difficult it is to tease out the reality and legalities of the situation. Sexual harassment incidents belong to this category as it causes deep anguish and is intertwined with interpersonal conflict. An example of such a case discussed below shows how convoluted the situation could become:
A female account director at a medical products company made a sexual harassment complaint about an employee. The employee was investigated and fired but given a substantial severance package. The female director went on to have some conduct issues with the company and was subsequently fired. However, she was not given any severance payout. A complicated and extracted court case followed. The result was that the ex-account director was able to sue the company under “Title VII of the Civil Rights Act of 1964” stating the company had retaliated against her in response to the internal complaint. The female ex-account director won her case.
In this instance, having the ability to monitor employees would have helped to prevent a retaliation case holding weight. AI-enabled employee monitoring would have alerted the company about issues before they turned more serious. If the case still progressed, during an employee investigation, interrogation of data by an AI-enabled platform would have provided vital evidence to help establish the facts.
Taking data for granted: Non-compete agreement breaches
Non-compete clauses help to protect sensitive data and intellectual property (IP). However, they can also be very difficult to defend. There are several ways to upheld non-compete agreements but all require court intervention. Court rulings involve injunctive relief and monetary damages paid. The latter requires very strong evidence of malicious conduct.
The court extract of a damages claim case involving Gregory Deger, a former employee of Cambridge Engineering, Inc. (Cambridge) who switched job to a new employer Mercury Partners 90 BI, Inc. repeatedly points out the lack of adequate evidence. In conclusion, the case states:
“There was no competent evidence which could establish that the Claimant [Deger] willfully and deliberately violated any company rule or policy of the Employer or that the claimant’s actions caused harm to the employer.”
AI-enabled employee investigations can harness data to provide the necessary evidence for proving similar cases clearly and compellingly.
The oppressor: Bullying
Being bullied in the workplace is a very serious accusation. It is damaging to the bullied but could equally hurt the accused if the accusation is false. A Workplace Bullying Institute research reported 19% of U.S. employees to experience bullying in the workplace. 65% of those are female. Bullying is another interpersonal issue that is complicated to prove or disprove. Bullying can take many forms and can be insidious and cause toxic relationships that cause workplace disruption and affect productivity.
Employee monitoring can nip bullying in the bud and help build bridges. However, such monitoring must be done in an empathetic and non-intrusive manner. AI-enabled employee monitoring platforms are designed to work in a non-intrusive manner.
Hardcore: Violence in the workplace
In 2018, in the USA there were 20,790 reported injuries caused by assaults in the workplace with 453 fatalities. Investigating claims of violence is a complicated and often drawn-out process that requires lawful proceedings. However, violent actions usually follow prior warning signs. Pre-emptive policy to prevent violence in the workplace relies on proper understanding and evaluation of these warnings.
Employee monitoring tools can be used unobtrusively to provide an early warning system before a tragic event occurs.
Office perks: Theft of company property
The 2020 Report to the Nations survey found the average loss per case of occupational fraud and abuse is around $1.5 million. Theft of company property is not just about money. Losses due to non-cash theft of corporate property cover 18% of all losses. Monitoring employees can help with the detection, response, and investigation of corporate asset theft.
Workplace situations can be delicate and convoluted. A company must tread carefully when investigating claims and misconduct. However, a Harris Poll shows that employees are open to employee monitoring. The poll found that 64% of Americans are OK with an employer monitoring digital activity as long as they are transparent about it.
Workplace investigations and the challenge of big data
The act of investigating your workforce brings many ethical issues into play. The process must be carried out respectfully and diligently. At the same time, care must be taken to collect timely and verifiable evidence. This delicate balance comes with many challenges.
Large amounts of data
Today, most companies are digital by default. Digitized processes produce massive amounts of data from emails, IoT, apps (both mobile and web). These data are often highly dispersed across multiple IT Infrastructures.
Disparate sources of unstructured data
Data comes in many forms. Enterprise data include scanned hard-copy documents, emails, apps, web apps, corporate and personal apps, messaging systems, digital assistants, etc. As much as 80% of this data is unstructured. Getting insights from unstructured data can be difficult. Unstructured data is inherently organic and contains ‘noise’ that makes patterns, signals, indicators, and events difficult to decipher.
Another challenge is with data being generated across multiple platforms, often based in the cloud. This mix of unstructured, disparate data creates a difficult data environment to navigate the level of insight needed to investigate workplace issues.
AI and the subset methodology, machine learning (ML) derive meaning from unstructured data across disparate sources.
Skills gap
Compounding the issue of massive amounts of disparate data is a lack of skills to deal with it. The data scientist skills gap is expected to present a global tech talent shortage of 85 million by 2030.
Ultimately, there is now so much data that human operators alone cannot handle it. An approach to data discovery and analysis based on artificial intelligence (AI) augments and enhances workplace investigations. AI effectively allows an organization to find a needle in a corporate data haystack.
Using AI to augment your workplace investigations
AI is being applied to employee monitoring and employee investigations across multiple industries from healthcare to police work. In policing, it is used to build intelligent insights using very large amounts of structured and (importantly) unstructured data. AI-enabled investigations, along with advanced analytics, use smart pattern recognition to look for unusual behavior and anomalies that police officers can use to build a case. Similarly, in the workplace, AI can augment employee investigations. AI does the leg work by analyzing large amounts of seemingly unconnected data to find patterns. AI is put to work to evaluate and classify data. The consequent data-derived intelligence is used to flag anomalies and spot the tell-tale signs of suspicious behavior.
Types of data used in AI-enabled workplace Investigations
Data across all spheres of working life can be used in AI-enabled employee monitoring and workplace investigations. Relevant data includes both structured and unstructured data from social networks, IoT devices, emails, collaboration platforms (e.g. Slack), and corporate and personal mobile devices.
Other data such as bank account records may also be relevant to a case but may require additional consent.
AI can help in employee monitoring and workplace investigations
In modern workplaces, employee monitoring and workplace investigations are the main areas that benefit from the empowering nature of AI-based analytics.
Employee Monitoring
Employee monitoring is an example of prevention being better than a cure. Being able to spot issues as they appear or before they build-up, prevents employee behavioral issues from becoming serious disputes.
One of the problems inherent in employee monitoring is the sensitive nature of the data involved. However, by choosing the right AI-enabled platforms, monitoring does not have to be intrusive and can become part of workplace culture.
Employee monitoring tools include User and Entity Behavioral Analysis (UEBA) and User Activity Monitoring (UAM). These tools monitor network events to look for anomalous behaviors. UEBA works from a baseline of expected behavior. It then applies AI methods, such as machine learning to spot changes in normalized patterns of behavior as humans, devices, and networks interact. If an unusual event occurs, the software sends out an alert.
UEBA, coupled with UAM solutions, is applicable in situations that are sensitive and complex, such as workplace issues.
Employee investigations
If employee-related problems end up in a dispute, a company needs robust and thorough methods of investigation. Monitoring provides a foundation of information that can be used in employee investigations. However, monitoring should be augmented by intelligent analytics to add weight to an investigation. One of the best outcomes from an employee investigation is the mitigation of repeat offenses. Employee investigations can provide the intelligence needed to learn lessons from incidents so that structures and policies can be put in place to prevent them in the future.
Veriato Investigator is an easy-to-implement system that unobtrusively records employee activity. The data can be used to enable fast, accurate, and efficient employee investigations.
AI-enabled employee monitoring to protect against retaliation
Applying AI-based employee monitoring and investigation solutions help to prevent retaliation lawsuits. A recent study exemplifies how deep insights into employee behavior can prevent retaliation. The researchers point out that 44% of employees feel uneasy, making managers aware of misconduct, stating that “if they reported misconduct to management, they experienced retaliation”.
AI-enabled monitoring provides the data to identify and alert on misconduct events. This removes the need for employees to intervene, creating a degree of separation that protects employees. As a system for investigations, AI provides the means to prove a misconduct case, and in doing so, it protects both employees and the company.
Conclusion
AI is an important tool in augmenting employee investigations and employee monitoring in an age of massive, widely distributed data. Employee data may often be unstructured, but it is also highly informative. Fixing this mix to provide actionable insights demands smart technology. AI/ML is unique in the ability to drill down into data, even across disparate applications. But employee relations are complicated and sensitive, and legal and regulatory compliance adds a layer of complexity.
AI tools such as UEBA and UAM offers an enterprise a means to unobtrusively monitor employees while minimizing commercial disruption. These smart tools provide a company with data analysis to perform a focused and effective investigation. An investigation, done well, ensures that both employees and the business are protected. These tools are the modern way to be proactive in sticky situations in the workplace.
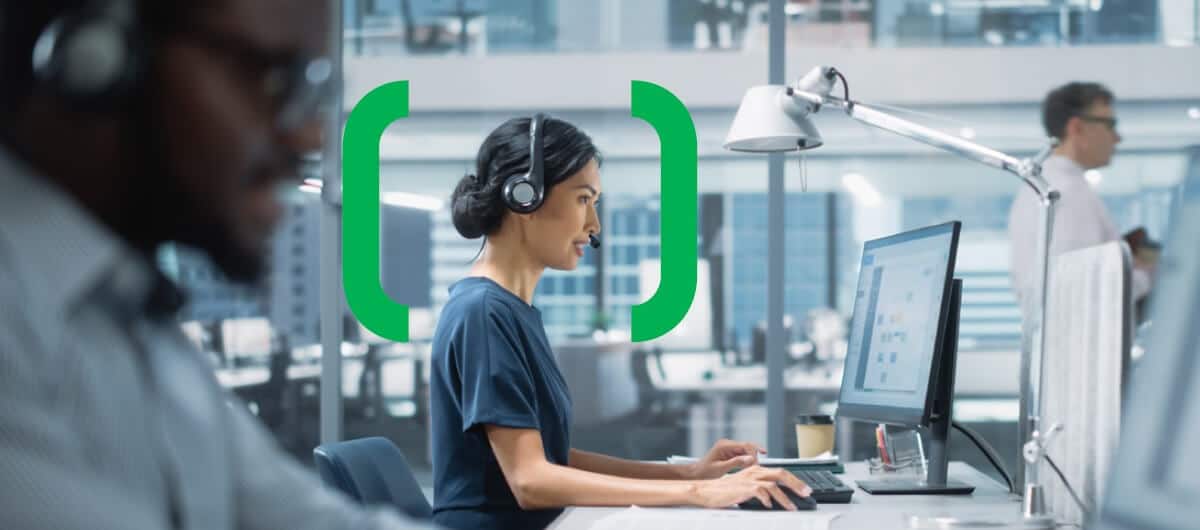
Insider Risk – How Prepared Are You?
Not every company is equally prepared to deal with insider risk. This report outlines the four stages of insider risk maturity and explores how to improve your insider risk preparedness.